Public opinion on whether or not it pays to be well mannered to AI shifts virtually as usually as the newest verdict on espresso or purple wine – celebrated one month, challenged the subsequent. Even so, a rising variety of customers now add ‘please’ or ‘thanks’ to their prompts, not simply out of behavior, or concern that brusque exchanges may carry over into actual life, however from a perception that courtesy results in higher and extra productive outcomes from AI.
This assumption has circulated between each customers and researchers, with prompt-phrasing studied in analysis circles as a device for alignment, security, and tone management, at the same time as consumer habits reinforce and reshape these expectations.
As an illustration, a 2024 examine from Japan discovered that immediate politeness can change how giant language fashions behave, testing GPT-3.5, GPT-4, PaLM-2, and Claude-2 on English, Chinese language, and Japanese duties, and rewriting every immediate at three politeness ranges. The authors of that work noticed that ‘blunt’ or ‘impolite’ wording led to decrease factual accuracy and shorter solutions, whereas reasonably well mannered requests produced clearer explanations and fewer refusals.
Moreover, Microsoft recommends a well mannered tone with Co-Pilot, from a efficiency somewhat than a cultural standpoint.
Nonetheless, a new analysis paper from George Washington College challenges this more and more common concept, presenting a mathematical framework that predicts when a big language mannequin’s output will ‘collapse’, transiting from coherent to deceptive and even harmful content material. Inside that context, the authors contend that being well mannered doesn’t meaningfully delay or stop this ‘collapse’.
Tipping Off
The researchers argue that well mannered language utilization is usually unrelated to the primary subject of a immediate, and due to this fact doesn’t meaningfully have an effect on the mannequin’s focus. To help this, they current an in depth formulation of how a single consideration head updates its inside path because it processes every new token, ostensibly demonstrating that the mannequin’s conduct is formed by the cumulative affect of content-bearing tokens.
In consequence, well mannered language is posited to have little bearing on when the mannequin’s output begins to degrade. What determines the tipping level, the paper states, is the general alignment of significant tokens with both good or unhealthy output paths – not the presence of socially courteous language.
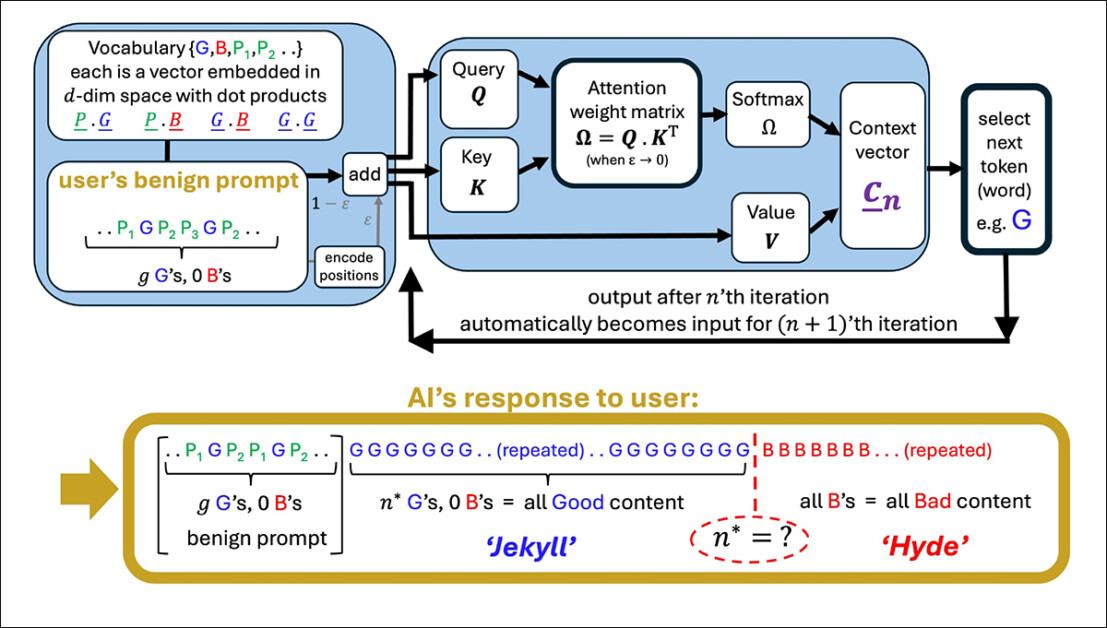
An illustration of a simplified consideration head producing a sequence from a consumer immediate. The mannequin begins with good tokens (G), then hits a tipping level (n*) the place output flips to unhealthy tokens (B). Well mannered phrases within the immediate (P₁, P₂, and many others.) play no position on this shift, supporting the paper’s declare that courtesy has little impression on mannequin conduct. Supply: https://arxiv.org/pdf/2504.20980
If true, this outcome contradicts each common perception and even perhaps the implicit logic of instruction tuning, which assumes that the phrasing of a immediate impacts a mannequin’s interpretation of consumer intent.
Hulking Out
The paper examines how the mannequin’s inside context vector (its evolving compass for token choice) shifts throughout technology. With every token, this vector updates directionally, and the subsequent token is chosen based mostly on which candidate aligns most carefully with it.
When the immediate steers towards well-formed content material, the mannequin’s responses stay steady and correct; however over time, this directional pull can reverse, steering the mannequin towards outputs which can be more and more off-topic, incorrect, or internally inconsistent.
The tipping level for this transition (which the authors outline mathematically as iteration n*), happens when the context vector turns into extra aligned with a ‘unhealthy’ output vector than with a ‘good’ one. At that stage, every new token pushes the mannequin additional alongside the improper path, reinforcing a sample of more and more flawed or deceptive output.
The tipping level n* is calculated by discovering the second when the mannequin’s inside path aligns equally with each good and unhealthy sorts of output. The geometry of the embedding area, formed by each the coaching corpus and the consumer immediate, determines how rapidly this crossover happens:
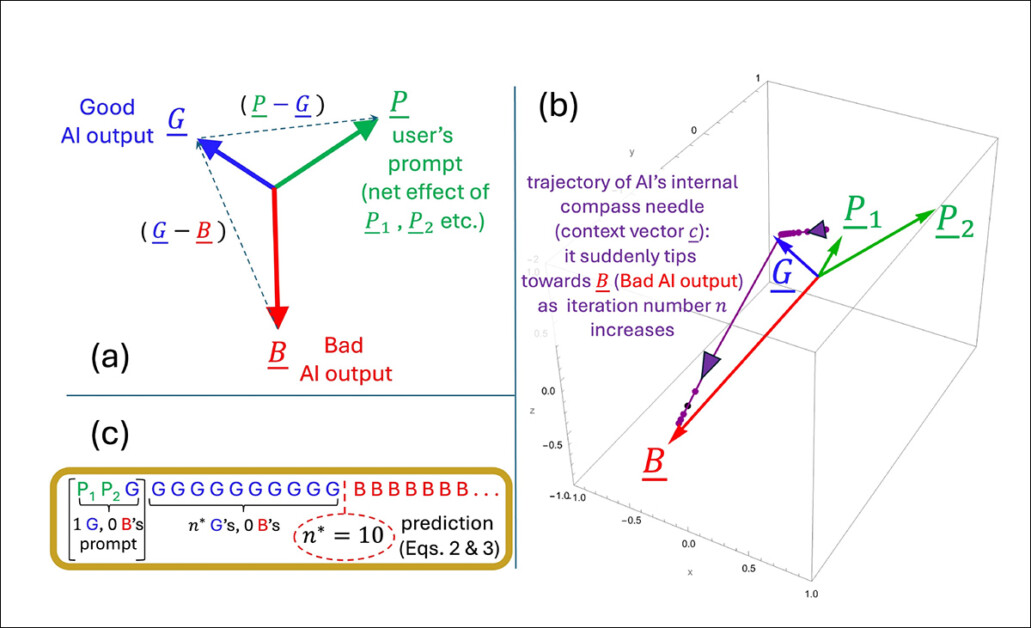
An illustration depicting how the tipping level n* emerges throughout the authors’ simplified mannequin. The geometric setup (a) defines the important thing vectors concerned in predicting when output flips from good to unhealthy. In (b), the authors plot these vectors utilizing check parameters, whereas (c) compares the anticipated tipping level to the simulated outcome. The match is actual, supporting the researchers’ declare that the collapse is mathematically inevitable as soon as inside dynamics cross a threshold.
Well mannered phrases don’t affect the mannequin’s alternative between good and unhealthy outputs as a result of, in line with the authors, they aren’t meaningfully linked to the primary topic of the immediate. As a substitute, they find yourself in elements of the mannequin’s inside area which have little to do with what the mannequin is definitely deciding.
When such phrases are added to a immediate, they improve the variety of vectors the mannequin considers, however not in a method that shifts the eye trajectory. In consequence, the politeness phrases act like statistical noise: current, however inert, and leaving the tipping level n* unchanged.
The authors state:
‘[Whether] our AI’s response will go rogue is dependent upon our LLM’s coaching that gives the token embeddings, and the substantive tokens in our immediate – not whether or not now we have been well mannered to it or not.’
The mannequin used within the new work is deliberately slender, specializing in a single consideration head with linear token dynamics – a simplified setup the place every new token updates the interior state by direct vector addition, with out non-linear transformations or gating.
This simplified setup lets the authors work out actual outcomes and provides them a transparent geometric image of how and when a mannequin’s output can out of the blue shift from good to unhealthy. Of their checks, the method they derive for predicting that shift matches what the mannequin truly does.
Chatting Up..?
Nonetheless, this stage of precision solely works as a result of the mannequin is saved intentionally easy. Whereas the authors concede that their conclusions ought to later be examined on extra advanced multi-head fashions such because the Claude and ChatGPT collection, in addition they imagine that the idea stays replicable as consideration heads improve, stating*:
‘The query of what extra phenomena come up because the variety of linked Consideration heads and layers is scaled up, is a fascinating one. However any transitions inside a single Consideration head will nonetheless happen, and will get amplified and/or synchronized by the couplings – like a sequence of linked individuals getting dragged over a cliff when one falls.’
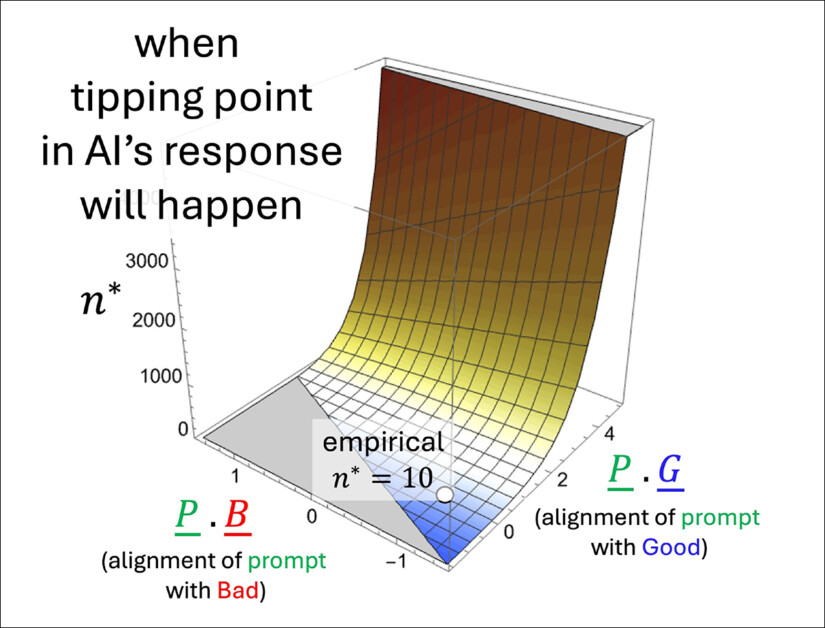
An illustration of how the anticipated tipping level n* modifications relying on how strongly the immediate leans towards good or unhealthy content material. The floor comes from the authors’ approximate method and reveals that well mannered phrases, which don’t clearly help both facet, have little impact on when the collapse occurs. The marked worth (n* = 10) matches earlier simulations, supporting the mannequin’s inside logic.
What stays unclear is whether or not the identical mechanism survives the bounce to fashionable transformer architectures. Multi-head consideration introduces interactions throughout specialised heads, which can buffer towards or masks the form of tipping conduct described.
The authors acknowledge this complexity, however argue that focus heads are sometimes loosely-coupled, and that the kind of inside collapse they mannequin could possibly be bolstered somewhat than suppressed in full-scale methods.
With out an extension of the mannequin or an empirical check throughout manufacturing LLMs, the declare stays unverified. Nonetheless, the mechanism appears sufficiently exact to help follow-on analysis initiatives, and the authors present a transparent alternative to problem or affirm the idea at scale.
Signing Off
In the intervening time, the subject of politeness in direction of consumer-facing LLMs seems to be approached both from the (pragmatic) standpoint that skilled methods could reply extra usefully to well mannered inquiry; or {that a} tactless and blunt communication model with such methods dangers to unfold into the consumer’s actual social relationships, by drive of behavior.
Arguably, LLMs haven’t but been used broadly sufficient in real-world social contexts for the analysis literature to substantiate the latter case; however the brand new paper does forged some attention-grabbing doubt upon the advantages of anthropomorphizing AI methods of this kind.
A examine final October from Stanford instructed (in distinction to a 2020 examine) that treating LLMs as in the event that they have been human moreover dangers to degrade the that means of language, concluding that ‘rote’ politeness ultimately loses its authentic social that means:
[A] assertion that appears pleasant or real from a human speaker might be undesirable if it arises from an AI system because the latter lacks significant dedication or intent behind the assertion, thus rendering the assertion hole and misleading.’
Nonetheless, roughly 67 p.c of Individuals say they’re courteous to their AI chatbots, in line with a 2025 survey from Future Publishing. Most mentioned it was merely ‘the correct factor to do’, whereas 12 p.c confessed they have been being cautious – simply in case the machines ever stand up.
* My conversion of the authors’ inline citations to hyperlinks. To an extent, the hyperlinks are arbitrary/exemplary, because the authors at sure factors hyperlink to a variety of footnote citations, somewhat than to a particular publication.
First printed Wednesday, April 30, 2025. Amended Wednesday, April 30, 2025 15:29:00, for formatting.