Qwen simply launched 8 new fashions as a part of its newest household – Qwen3, showcasing promising capabilities. The flagship mannequin, Qwen3-235B-A22B, outperformed most different fashions together with DeepSeek-R1, OpenAI’s o1, o3-mini, Grok 3, and Gemini 2.5-Professional, in commonplace benchmarks. In the meantime, the small Qwen3-30B-A3B outperformed QWQ-32B which has roughly 10 occasions the activated parameters as the brand new mannequin. With such superior capabilities, these fashions show to be an awesome alternative for a variety of purposes. On this article, we are going to discover the options of all of the Qwen3 fashions and discover ways to use them to construct RAG techniques and AI brokers.
What’s Qwen3?
Qwen3 is the most recent sequence of massive language fashions (LLMs) within the Qwen household, consisting of 8 totally different fashions. These embody Qwen3-235B-A22B, Qwen3-30B-A3B, Qwen3-32B, Qwen3-14B, Qwen3-8B, Qwen3-4B, Qwen3-1.7B, and Qwen3-0.6B. All these fashions are launched underneath Apache 2.0 license, making them freely obtainable to people, builders, and enterprises.
Whereas 6 of those fashions are dense, which means they actively use all of the parameters in the course of the time of inference and coaching, 2 of them are open-weighted:
- Qwen3-235B-A22B: A big mannequin with 235 billion parameters, out of which 22 billion are activated parameters.
- Qwen3-30B-A3B: A smaller MoE with 30 billion whole parameters and three billion activated parameters.
Right here’s an in depth comparability of all of the 8 Qwen3 fashions:
Fashions | Layers | Heads (Q/KV) | Tie Embedding | Context Size |
Qwen3-0.6B | 28 | 16/8 | Sure | 32K |
Qwen3-1.7B | 28 | 16/8 | Sure | 32K |
Qwen3-4B | 36 | 32/8 | Sure | 32K |
Qwen3-8B | 36 | 32/8 | No | 128K |
Qwen3-14B | 40 | 40/8 | No | 128K |
Qwen3-32B | 64 | 64/8 | No | 128K |
Qwen3-30B-A3B | 48 | 32/4 | No | 128K |
Qwen3-235B-A22B | 94 | 64/4 | No | 128K |
Right here’s what the desk says:
- Layers: Layers signify the variety of transformer blocks used. It consists of multi-head self-attention mechanism, feed ahead networks, positional encoding, layer normalization, and residual connections. So, after I say Qwen3-30B-A3B has 48 layers, it implies that the mannequin makes use of 48 transformer blocks, stacked sequentially or in parallel.
- Heads: Transformers use multi-head consideration, which splits its consideration mechanism into a number of heads, every for studying a brand new side from the information. Right here, Q/KV represents:
- Q (Question heads): Complete variety of consideration heads used for producing queries.
- KV (Key and Worth): The variety of key/worth heads per consideration block.
Be aware: These consideration heads for Key, Question, and Worth are utterly totally different from the important thing, question, and worth vector generated by a self-attention.
Additionally Learn: Qwen3 Fashions: Learn how to Entry, Efficiency, Options, and Purposes
Key Options of Qwen3
Listed here are a few of the key options of the Qwen3 fashions:
- Pre-training: The pre-training course of consists of three phases:
- Within the first stage, the mannequin was pretrained on over 30 trillion tokens with a context size of 4k tokens. This taught the mannequin fundamental language expertise and common data.
- Within the second stage, the standard of knowledge was improved by growing the proportion of knowledge-intensive knowledge like STEM, coding, and reasoning duties. The mannequin was then skilled over a further 5 trillion tokens.
- Within the remaining stage, top quality lengthy context knowledge was utilized by growing the context size to 32K tokens. This was completed to make sure that the mannequin can deal with longer inputs successfully.
- Publish-training: To develop a hybrid mannequin able to each step-by-step reasoning and fast responses, a 4-stage coaching pipeline was carried out. This consisted of:
- Hybrid Considering Modes: Qwen3 fashions use a hybrid strategy to downside fixing, that includes two new modes:
- Considering Mode: On this mode, fashions take time by breaking a fancy downside assertion into small and procedural steps to unravel it.
- Non-Considering Mode: On this mode, the mannequin supplies fast outcomes and is usually appropriate for easier questions.
- Multilingual Help: Qwen3 fashions help 119 languages and dialects. This helps customers from all world wide to learn from these fashions.
- Improvised Agentic Capabilities: Qwen has optimized the Qwen3 fashions for higher coding and agentic capabilities, supporting Mannequin Context Protocol (MCP) as nicely.
Learn how to Entry Qwen3 Fashions by way of API
To make use of the Qwen3 fashions, we will probably be accessing it by way of API utilizing the Openrouter API. Right here’s tips on how to do it:
- Create an account on Openrouter and go to the mannequin search bar to search out the API for that mannequin.
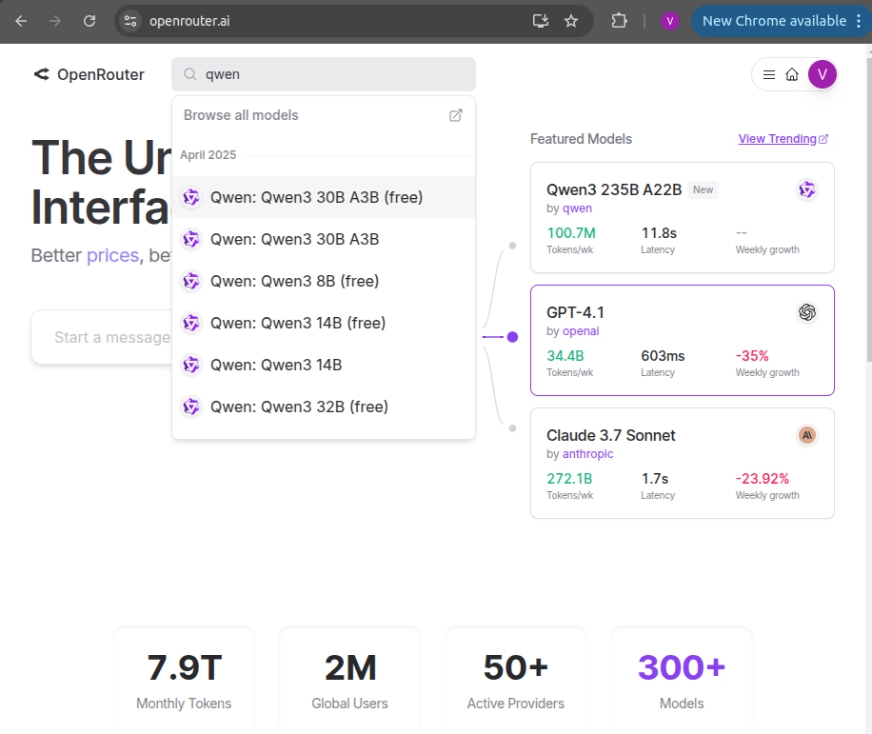
- Choose the mannequin of your alternative and click on on ‘Create API key’ on the touchdown web page to generate a brand new API.
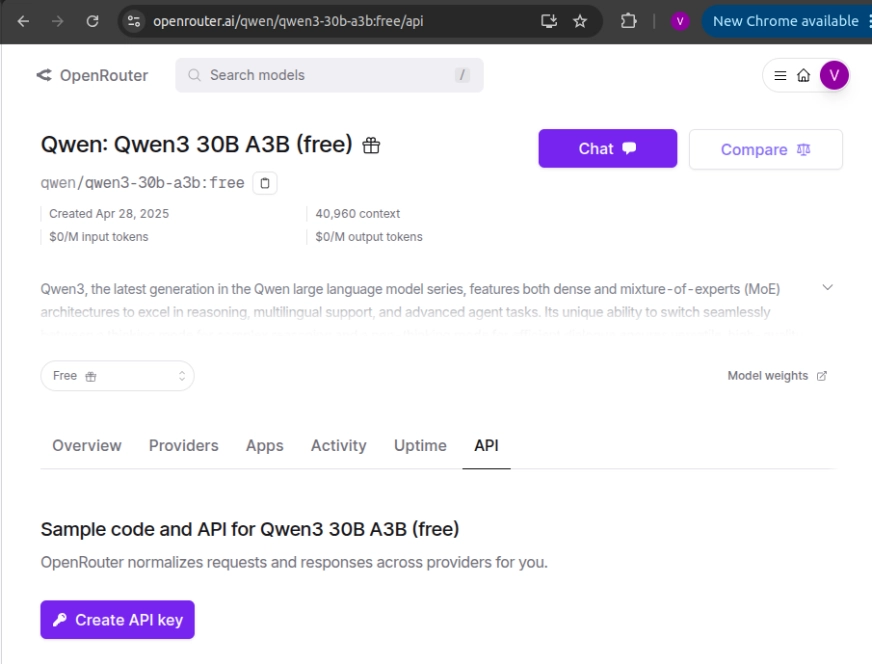
Utilizing Qwen3 to Energy Your AI Options
On this part, we’ll undergo the method of constructing AI purposes utilizing Qwen3. We are going to first create an AI-powered journey planner agent utilizing the mannequin, after which a Q/A RAG bot utilizing Langchain.
Stipulations
Earlier than constructing some real-world AI options with Qwen3, we have to first cowl the fundamental conditions like:
Constructing an AI Agent utilizing Qwen3
On this part, we’ll be utilizing Qwen3 to create an AI-powered journey agent that may give the key touring spots for town or place you might be visiting. We may even allow the agent to look the web to search out up to date data, and add a software that permits foreign money conversion.
Step 1: Organising Libraries and Instruments
First, we will probably be putting in and importing the mandatory libraries and instruments required to construct the agent.
!pip set up langchain langchain-community openai duckduckgo-search
from langchain.chat_models import ChatOpenAI
from langchain.brokers import Instrument
from langchain.instruments import DuckDuckGoSearchRun
from langchain.brokers import initialize_agent
llm = ChatOpenAI(
base_url="https://openrouter.ai/api/v1",
api_key="your_api_key",
mannequin="qwen/qwen3-235b-a22b:free"
)
# Internet Search Instrument
search = DuckDuckGoSearchRun()
# Instrument for DestinationAgent
def get_destinations(vacation spot):
return search.run(f"High 3 vacationer spots in {vacation spot}")
DestinationTool = Instrument(
identify="Vacation spot Recommender",
func=get_destinations,
description="Finds high locations to go to in a metropolis"
)
# Instrument for CurrencyAgent
def convert_usd_to_inr(question):
quantity = [float(s) for s in query.split() if s.replace('.', '', 1).isdigit()]
if quantity:
return f"{quantity[0]} USD = {quantity[0] * 83.2:.2f} INR"
return "Could not parse quantity."
CurrencyTool = Instrument(
identify="Forex Converter",
func=convert_usd_to_inr,
description="Converts USD to inr based mostly on static charge"
)
- Search_tool: DuckDuckGoSearchRun() permits the agent to make use of internet search to get real-time details about the favored vacationer spots.
- DestinationTool: Applies the get_destinations() operate, which makes use of the search software to get the highest 3 vacationer spots in any given metropolis.
- CurrencyTool: Makes use of the convert_usd_to_inr() operate to transform the costs from USD to INR. You’ll be able to change ‘inr’ within the operate to transform it to a foreign money of your alternative.
Additionally Learn: Construct a Journey Assistant Chatbot with HuggingFace, LangChain, and MistralAI
Step 2: Creating the Agent
Now that we have now initialized all of the instruments, let’s proceed to creating an agent that may use the instruments and provides us a plan for the journey.
instruments = [DestinationTool, CurrencyTool]
agent = initialize_agent(
instruments=instruments,
llm=llm,
agent_type="zero-shot-react-description",
verbose=True
)
def trip_planner(metropolis, usd_budget):
dest = get_destinations(metropolis)
inr_budget = convert_usd_to_inr(f"{usd_budget} USD to INR")
return f"""Right here is your journey plan:
*High spots in {metropolis}*:
{dest}
*Price range*:
{inr_budget}
Take pleasure in your day journey!"""
- Initialize_agent: This operate creates an agent with Langchain utilizing a zero-shot response strategy, which permits the agent to grasp the software descriptions.
- Agent_type: “zero-shot-react-description” permits the agent LLM to resolve which software it ought to use in a sure scenario with out prior data, through the use of the software description and enter.
- Verbose: Verbose permits the logging of the agent’s thought course of, so we will monitor every determination that the agent makes, together with all of the interactions and instruments invoked.
- trip_planner: It is a python operate that manually calls instruments as an alternative of counting on the agent. It permits the person to pick out the perfect software for a selected downside.
Step 3: Initializing the Agent
On this part, we’ll be initializing the agent and observing its response.
# Initialize the Agent
metropolis = "Delhi"
usd_budget = 8500
# Run the multi-agent planner
response = agent.run(f"Plan a day journey to {metropolis} with a finances of {usd_budget} USD")
from IPython.show import Markdown, show
show(Markdown(response))
- Invocation of agent: agent.run() makes use of the person’s intent by way of immediate and plans the journey.
Output
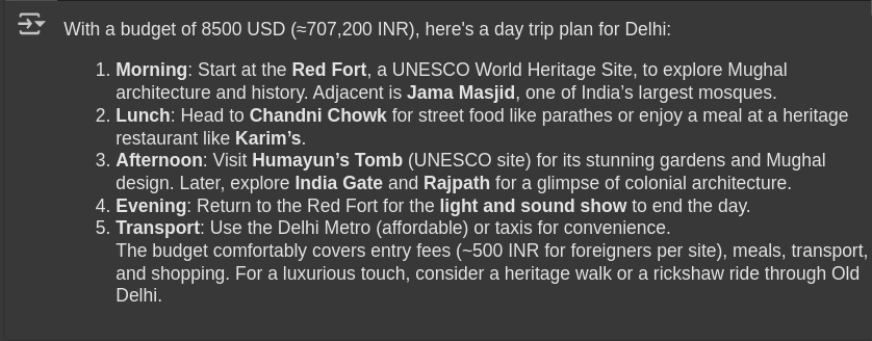
Constructing a RAG System utilizing Qwen3
On this part, we’ll be making a RAG bot that solutions any question throughout the related enter doc from the data base. This provides an informative response utilizing qwen/qwen3-235b-a22b. The system would even be utilizing Langchain, to supply correct and context-aware responses.
Step 1: Organising the Libraries and Instruments
First, we will probably be putting in and importing the mandatory libraries and instruments required to construct the RAG system.
!pip set up langchain langchain-community langchain-core openai tiktoken chromadb sentence-transformers duckduckgo-search
from langchain_community.document_loaders import TextLoader
from langchain.text_splitter import CharacterTextSplitter
from langchain_community.vectorstores import Chroma
from langchain.embeddings import HuggingFaceEmbeddings
from langchain.chains import RetrievalQA
from langchain.chat_models import ChatOpenAI
# Load your doc
loader = TextLoader("/content material/my_docs.txt")
docs = loader.load()
- Loading Paperwork: The “TextLoader” class of Langchain hundreds the doc like a pdf, txt, or doc file which will probably be used for the Q/A retrieval. Right here I’ve uploaded my_docs.txt.
- Deciding on the Vector Setup: I’ve used ChromaDB to retailer and search the embeddings from our vector database for the Q/A course of.
Step 2: Creating the Embeddings
Now that we’ve loaded our doc, let’s proceed to creating embeddings out of it which can assist in easing the retrieval course of.
# Break up into chunks
splitter = CharacterTextSplitter(chunk_size=300, chunk_overlap=50)
chunks = splitter.split_documents(docs)
# Embed with HuggingFace mannequin
embeddings = HuggingFaceEmbeddings(model_name="all-MiniLM-L6-v2")
db = Chroma.from_documents(chunks, embedding=embeddings)
# Setup Qwen LLM from OpenRouter
llm = ChatOpenAI(
base_url="https://openrouter.ai/api/v1",
api_key="YOUR_API_KEY",
mannequin="qwen/qwen3-235b-a22b:free"
)
# Create RAG chain
retriever = db.as_retriever(search_kwargs={"ok": 2})
rag_chain = RetrievalQA.from_chain_type(llm=llm, retriever=retriever)
- Doc Splitting: The CharacterTextSplitter() splits the textual content into smaller chunks, which can primarily assist in two issues. First, it eases the retrieval course of, and second, it helps in retaining the context from the earlier chunk by way of chunk_overlap.
- Embedding Paperwork: Embeddings convert the textual content into the embedding vectors of a set dimension for every token. Right here we have now used chunk_size of 300, which suggests each phrase/token will probably be transformed right into a vector of 300 dimensions. Now this vector embedding can have all of the contextual data of that phrase with respect to the opposite phrases within the chunk.
- RAG Chain: RAG chain combines the ChromaDB with the LLM to type a RAG. This allows us to get contextually conscious solutions from the doc in addition to from the mannequin.
Step 3: Initializing the RAG System
# Ask a query
response = rag_chain.invoke({"question": "How can i take advantage of Qwen with MCP. Please give me a stepwise information together with the mandatory code snippets"})
show(Markdown(response['result']))
- Question Execution: The rag_chain_invoke() technique will ship the person’s question to the RAG system, which then retrieves the related context-aware chunks from the doc retailer (vector db) and generates a context-aware reply.
Output
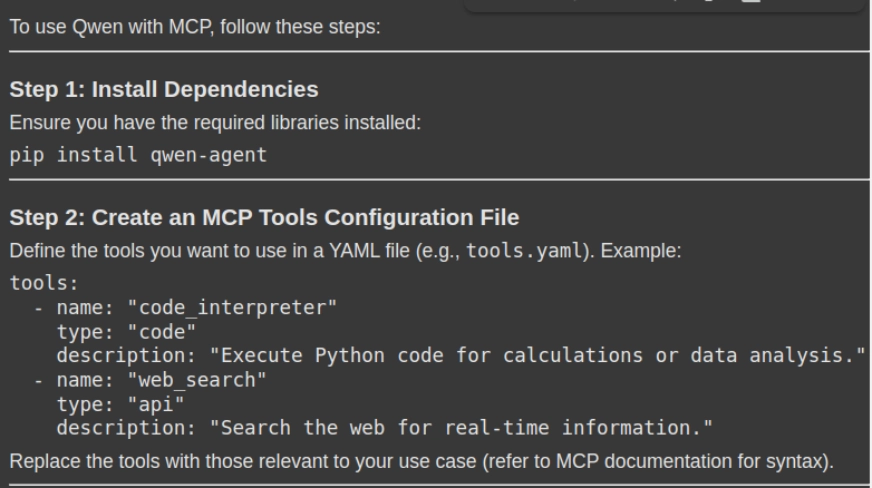
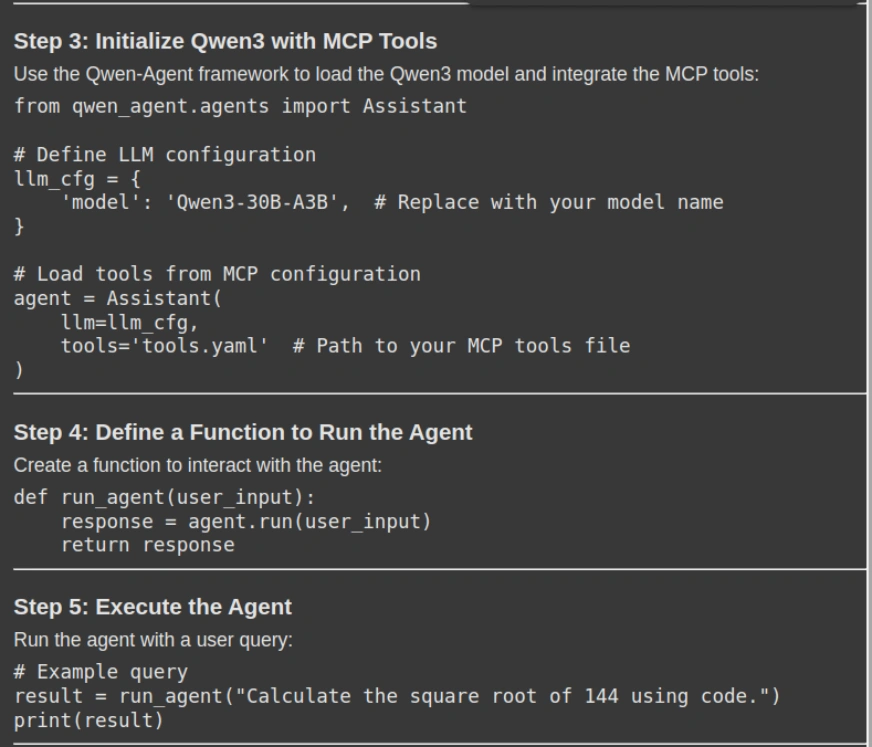
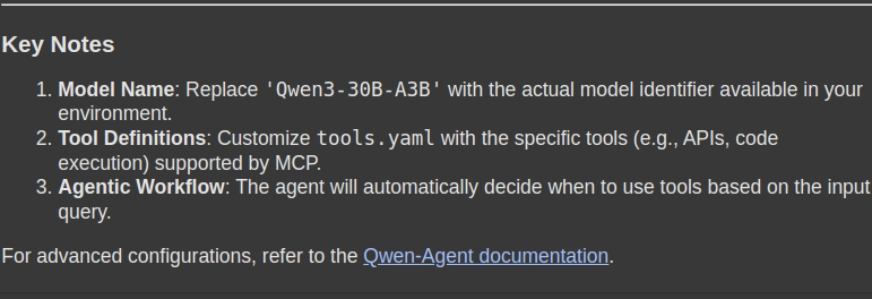
You’ll find the entire code right here.
Purposes of Qwen3
Listed here are some extra purposes of Qwen3 throughout industries:
- Automated Coding: Qwen3 can generate, debug, and supply documentation for code, which helps builders to unravel errors with out guide effort. Its 22B parameter mannequin excels in coding, with performances similar to fashions like DeepSeek-R1, Gemini 2.5 Professional, and OpenAI’s o3-mini.
- Schooling and Analysis: Qwen3 archives excessive accuracy in math, physics, and logical reasoning downside fixing. It additionally rivals the Gemini 2.5 Professional, whereas excels with fashions resembling OpenAI’s o1, o3-mini, DeepSeek-R1, and Grok 3 Beta.
- Agent-Based mostly Instrument Integration: Qwen3 additionally leads in AI agent duties by permitting using exterior instruments, APIs, and MCPs for multi-step and multi-agentic workflows with its tool-calling template, which additional simplifies the agentic interplay.
- Superior Reasoning Duties: Qwen3 makes use of an intensive considering functionality to ship optimum and correct responses. The mannequin makes use of chain-of-thought reasoning for complicated duties and a non-thinking mode for optimized velocity.
Conclusion
On this article, we have now discovered tips on how to construct Qwen3-powered agentic AI and RAG techniques. Qwen3’s excessive efficiency, multilingual help, and superior reasoning functionality make it a robust alternative for data retrieval and agent-based duties. By integrating Qwen3 into RAG and agentic pipelines, we will get correct, context-aware, and clean responses, making it a robust contender for real-world purposes for AI-powered techniques.
Regularly Requested Questions
A. Qwen3 has a hybrid reasoning functionality that enables it to make dynamic modifications within the responses, which permits it to optimize the RAG workflows for each retrieval and sophisticated evaluation.
A. It majorly consists of the Vector database, Embedding fashions, Langchain workflow and an API to entry the mannequin.
Sure, with the Qwen-agent built-in software calling templates, we will parse and allow sequential software operations like internet looking out, knowledge evaluation, and report technology.
A. One can scale back the latency in some ways, a few of them are:
1. Use of MOE fashions like Qwen3-30B-A3B, which solely have 3 billion lively parameters.
2. Through the use of GPU-optimized inferences.
A. The frequent error consists of:
1. MCP server initialization failures, like json formatting and INIT.
2. Instrument response pairing errors.
3. Context window overflow.
Login to proceed studying and luxuriate in expert-curated content material.