Efficiently implementing scientific AI can reshape the trajectory of a well being system — enhancing margins, energizing employees and accelerating higher outcomes — however when executed poorly, it dangers turning into simply one other overpromised answer that fails to ship significant change.
Nowhere is that threat extra evident than within the frequent confusion between AI platforms and AI marketplaces. On the floor, they will sound related. Each promise scale. Each declare integration. Nonetheless, beneath the advertising and marketing language are important variations that form how AI is deployed, ruled and scaled.
Understanding these variations isn’t only a technical distinction; it’s a strategic one. On this publish, we consider what units platforms and marketplaces aside, why confusion between the 2 persists and what’s at stake when well being programs wager on the incorrect AI integration strategy.
What’s a Scientific AI Platform?
A true scientific AI platform is an end-to-end, built-in system the place AI isn’t simply layered on prime of workflows — it’s embedded at each stage. Algorithms run natively throughout the infrastructure, repeatedly analyzing knowledge at scale. Outputs aren’t solely actionable but in addition measurable, with real-time suggestions loops that quantify affect and drive ongoing optimization throughout scientific, operational and monetary metrics.
A scientific AI platform gives 4 important features:
- Run AI (Standardized): Ingest and normalize messy knowledge, orchestrate logic and monitor efficiency in real-time.
- Drive Motion (Embedded): Ship outcomes by means of clinician-friendly interfaces built-in into native programs and linked throughout domains. For instance, linking a radiology workstation to a cellular app or care coordination software to make sure well timed follow-through.
- Measure Impression (Centralized): Monitor engagement, scientific outcomes and system-wide worth by means of a shared telemetry layer.
- Use Circumstances (Interoperable): Supply a rising catalog of AI purposes which are deployed inside a unified, scalable infrastructure.
With out the primary three layers, AI can’t function successfully at scale, and that’s the place platforms stand aside.
What’s a Market?
A market is a curated catalog of third-party AI instruments, providing entry to dozens of algorithms in a single place. Whereas this mannequin affords breadth, it lacks the infrastructure to assist enterprise-grade deployment at scale.
A market sometimes introduces 4 key challenges:
- Run AI (Decentralized): Every algorithm requires its personal integration pathway, knowledge mapping, orchestration logic and runtime atmosphere, with no unified solution to handle good orchestration throughout deployment or monitor efficiency afterward. This locations a heavy burden on IT groups, making it troublesome to deploy and keep a number of AI options at scale. In consequence, websites wrestle to make sure constant algorithm efficiency, decreasing scientific affect and resulting in missed alternatives to determine at-risk sufferers.
- Drive Motion (Disjointed): Outputs are delivered through standalone UIs or asynchronous programs that aren’t embedded inside native scientific workflows.
- Measure Impression (Non-Uniform): With out a shared telemetry layer or deep system integration, most marketplaces lack the flexibility to measure the affect of a single algorithm — making it practically unimaginable to trace actual utilization, consider efficiency or show outcomes in a constant, clinically significant manner.
- Use Circumstances (Siloed): Algorithms are deployed as level options with no shared providers layer, limiting interoperability and compounding operational overhead.
With out platform-level cohesion, marketplaces can place heavy burdens on IT, disrupt scientific workflows and inhibit system-wide adoption.
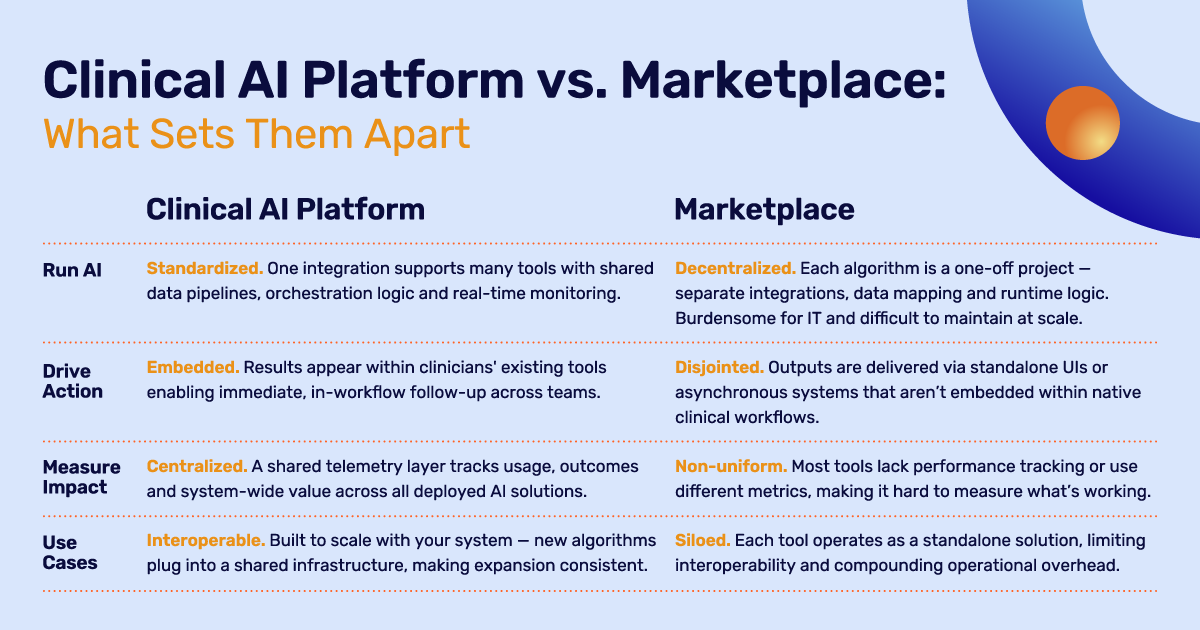
Why Are the Two Approaches Confused?
From the surface, platforms and marketplaces can seem related. Each promise entry to a number of AI options, however the similarities cease on the floor. The underlying infrastructure, workflow integration and scientific usability are basically completely different.
This confusion typically stems from restricted real-world expertise. For well being programs simply starting their AI journey, a big algorithm catalog can seem to be the quickest path to scale. Nonetheless, what’s typically missed is the operational complexity: every answer requires its personal integration, person interface and workflow governance and clinician coaching — none of which scales simply.
The one greatest distinction? A platform centralizes AI execution, motion and oversight, whereas a market externalizes it. One is constructed to scale with your well being system. The opposite asks your well being system to scale round it.
What’s the Threat of Complicated AI Platforms and AI Marketplaces?
Well being programs might imagine: “We’ll want AI for radiology, cardiology and Emergency Division (ED) triage, why not select a market with all of it?”
The issue is that almost all marketplaces lack each the infrastructure to scale and the instruments to run AI intelligently. Every algorithm typically requires its personal integration, safety evaluation, authorized settlement and workflow design, which limits rollout to only one or two instruments per 12 months.
Even after deployment, efficiency suffers. With out good orchestration, algorithms don’t run optimally on real-world knowledge, resulting in inconsistent outcomes and lowered scientific affect — figuring out fewer sufferers than they need to.
Usability is one other problem. Clinicians might have to change between interfaces, interpret outputs delivered on completely different timelines or seek for outcomes exterior of their native programs. This introduces friction as a substitute of effectivity.
Then there’s the ultimate problem: affect. With out a constant solution to measure outcomes throughout instruments, well being programs don’t have any clear view of what’s working, what’s not or the place to optimize.
Against this, a real scientific AI platform allows well being programs to deploy and scale dozens of purposes by means of a single, unified infrastructure, making AI simpler to undertake, govern and scale.
Easy methods to Consider a Associate
Complicated a market for a platform isn’t only a technical misstep — it’s a strategic one. The results ripple throughout scientific, operational and monetary efficiency. That’s why it’s important to look past surface-level claims and dig into what the underlying expertise truly delivers.
For those who’re evaluating AI to your well being system, don’t simply depend algorithms. Ask what’s beneath:
- What number of algorithms can I realistically deploy in 12 months?
- How a lot IT effort will that require?
- Do I’ve a single level of contact for each integration and ongoing assist?
- How are these algorithms built-in into scientific workflows — and is that workflow constant throughout all clinicians?
- Who owns the configuration and scientific supply of every software?
- Can we measure affect persistently throughout the system?
Platforms aren’t simply extra usable — they’re extra scalable, extra measurable and extra clinically sustainable. And that makes all of the distinction while you’re betting on enterprise-wide AI.